Discovering weather periods and crop properties favorable for coffee rust incidence from feature selection approaches
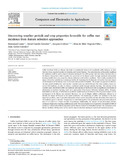
View/ Open
Date
2020Author
Lasso, Emmanuel
Corrales, David Camilo
Avelino, Jacques
Virginio Filho, Elias de Melo
Corrales, Juan Carlos
Type
Artículo
Metadata
Show full item recordAbstract
Coffee Leaf Rust (CLR) is a disease that leads to considerable losses in the worldwide coffee industry; as those that have been reported recently in Colombia and Central America. The early detection of favorable conditions for epidemics could be used to improve decision making for the coffee grower and thus reduce the losses due to the disease. Researchers tried to predict the occurrence of the disease earlier through statistical and machine learning models from crop properties, disease indicators and weather conditions. These studies considered the impact of weather variables in a common period for all. Assuming that the dynamics of weather that most impact the development of the disease occur in the same time periods is simplistic. We propose an approach to discover the time period (window) for each weather variables and crop related features that most explain a future observed CLR incidence, in order to obtain a prediction model through machine learning. The selection of the variables more related with coffee rust incidence and rejection of the features with no significant contribution of information in machine learning tasks were approached from Feature Selection methods (Filter, Wrapper, Embedded). In this way, a CLR incidence prediction model based on the features with the greatest impact on the development of the disease was obtained. Moreover, the use of SHapley Additive exPlanations allowed us to identify the impact of features in the model prediction...
Keywords
Is part of
Computers and Electronics in Agriculture
URI (Permanet link to cite or share this item)
https://doi.org/10.1016/j.compag.2020.105640https://repositorio.catie.ac.cr/handle/11554/10289